A mannequin’s capability to generalize is influenced by each the range of the information and the way in which the mannequin is educated, researchers report.
Synthetic intelligence methods might be able to full duties rapidly, however that doesn’t imply they at all times accomplish that pretty. If the datasets used to coach machine-learning fashions comprise biased information, it's probably the system may exhibit that very same bias when it makes selections in observe.
For example, if a dataset incorporates principally photographs of white males, then a facial-recognition mannequin educated with these information could also be much less correct for girls or individuals with completely different pores and skin tones.
A gaggle of researchers at MIT, in collaboration with researchers at Harvard College and Fujitsu Ltd., sought to know when and the way a machine-learning mannequin is able to overcoming this type of dataset bias. They used an strategy from neuroscience to check how coaching information impacts whether or not a synthetic neural community can be taught to acknowledge objects it has not seen earlier than. A neural community is a machine-learning mannequin that mimics the human mind in the way in which it incorporates layers of interconnected nodes, or “neurons,” that course of information.
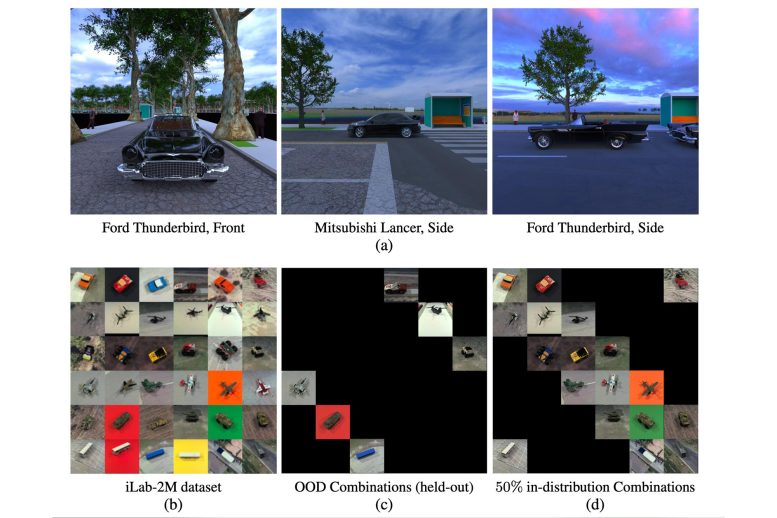
If researchers are coaching a mannequin to categorise vehicles in photographs, they need the mannequin to be taught what completely different vehicles seem like. But when each Ford Thunderbird within the coaching dataset is proven from the entrance, when the educated mannequin is given a picture of a Ford Thunderbird shot from the aspect, it could misclassify it, even when it was educated on hundreds of thousands of automobile photographs. Credit score: Picture courtesy of the researchers
The brand new outcomes present that range in coaching information has a serious affect on whether or not a neural community is ready to overcome bias, however on the similar time dataset range can degrade the community’s efficiency. In addition they present that how a neural community is educated, and the particular varieties of neurons that emerge through the coaching course of, can play a serious position in whether or not it is ready to overcome a biased dataset.
“A neural community can overcome dataset bias, which is encouraging. However the primary takeaway right here is that we have to have in mind information range. We have to cease pondering that when you simply acquire a ton of uncooked information, that's going to get you someplace. We must be very cautious about how we design datasets within the first place,” says Xavier Boix, a analysis scientist within the Division of Mind and Cognitive Sciences (BCS) and the Heart for Brains, Minds, and Machines (CBMM), and senior writer of the paper.
Co-authors embody former MIT graduate college students Timothy Henry, Jamell Dozier, Helen Ho, Nishchal Bhandari, and Spandan Madan, a corresponding writer who's at the moment pursuing a PhD at Harvard; Tomotake Sasaki, a former visiting scientist now a senior researcher at Fujitsu Analysis; Frédo Durand, a professor of electrical engineering and pc science at MIT and a member of the Laptop Science and Synthetic Intelligence Laboratory; and Hanspeter Pfister, the An Wang Professor of Laptop Science on the Harvard College of Enginering and Utilized Sciences. The analysis seems in the present day in Nature Machine Intelligence.
Pondering like a neuroscientist
Boix and his colleagues approached the issue of dataset bias by pondering like neuroscientists. In neuroscience, Boix explains, it is not uncommon to make use of managed datasets in experiments, which means a dataset through which the researchers know as a lot as potential in regards to the data it incorporates.
The staff constructed datasets that contained photographs of various objects in diversified poses, and thoroughly managed the combos so some datasets had extra range than others. On this case, a dataset had much less range if it incorporates extra photographs that present objects from just one viewpoint. A extra various dataset had extra photographs displaying objects from a number of viewpoints. Every dataset contained the identical variety of photographs.
The researchers used these rigorously constructed datasets to coach a neural community for picture classification, after which studied how effectively it was in a position to establish objects from viewpoints the community didn't see throughout coaching (referred to as an out-of-distribution mixture).
For instance, if researchers are coaching a mannequin to categorise vehicles in photographs, they need the mannequin to be taught what completely different vehicles seem like. But when each Ford Thunderbird within the coaching dataset is proven from the entrance, when the educated mannequin is given a picture of a Ford Thunderbird shot from the aspect, it could misclassify it, even when it was educated on hundreds of thousands of automobile photographs.
The researchers discovered that if the dataset is extra various — if extra photographs present objects from completely different viewpoints — the community is healthier in a position to generalize to new photographs or viewpoints. Information range is vital to overcoming bias, Boix says.
“However it's not like extra information range is at all times higher; there's a pressure right here. When the neural community will get higher at recognizing new issues it hasn’t seen, then it'll turn into more durable for it to acknowledge issues it has already seen,” he says.
Testing coaching strategies
The researchers additionally studied strategies for coaching the neural community.
In machine studying, it is not uncommon to coach a community to carry out a number of duties on the similar time. The concept is that if a relationship exists between the duties, the community will be taught to carry out every one higher if it learns them collectively.
However the researchers discovered the alternative to be true — a mannequin educated individually for every activity was in a position to overcome bias much better than a mannequin educated for each duties collectively.
“The outcomes had been actually placing. In reality, the primary time we did this experiment, we thought it was a bug. It took us a number of weeks to appreciate it was an actual end result as a result of it was so sudden,” he says.
They dove deeper contained in the neural networks to know why this happens.
They discovered that neuron specialization appears to play a serious position. When the neural community is educated to acknowledge objects in photographs, it seems that two varieties of neurons emerge — one that focuses on recognizing the article class and one other that focuses on recognizing the point of view.
When the community is educated to carry out duties individually, these specialised neurons are extra outstanding, Boix explains. But when a community is educated to do each duties concurrently, some neurons turn into diluted and don’t specialize for one activity. These unspecialized neurons usually tend to get confused, he says.
“However the subsequent query now's, how did these neurons get there? You practice the neural community they usually emerge from the educational course of. Nobody instructed the community to incorporate these kinds of neurons in its structure. That's the fascinating factor,” he says.
That's one space the researchers hope to discover with future work. They need to see if they'll power a neural community to develop neurons with this specialization. In addition they need to apply their strategy to extra advanced duties, comparable to objects with sophisticated textures or diversified illuminations.
Boix is inspired that a neural community can be taught to beat bias, and he's hopeful their work can encourage others to be extra considerate in regards to the datasets they're utilizing in AI purposes.
This work was supported, partly, by the Nationwide Science Basis, a Google School Analysis Award, the Toyota Analysis Institute, the Heart for Brains, Minds, and Machines, Fujitsu Analysis, and the MIT-Sensetime Alliance on Synthetic Intelligence.
Post a Comment